by Prof. Natalia Berloff, University of Cambridge
Diffusion models have revolutionized artificial intelligence, particularly in generative AI applications. These models excel at tasks such as image synthesis, audio generation, and video content creation, surpassing previous techniques like generative adversarial networks. Their ability to produce high-quality, diverse outputs has made them indispensable across various industries, from creative arts to scientific research. However, the computational demands of diffusion models pose significant challenges. The iterative process of adding and removing noise, central to these models, requires substantial processing power and energy consumption.
In machine learning, diffusion models generate data by iteratively refining noisy inputs. They operate through a two-stage process: a forward stage that gradually corrupts data with noise and a reverse stage that reconstructs the data from the noise. This iterative noise addition and subtraction is computationally intensive and energy-consuming, especially for high-resolution tasks.
Non-Hermitian optical systems, characterized by gain and dissipation, offer a rich platform for exploring unconventional behaviours in optics. These systems can amplify noise and exhibit asymmetric states from symmetric conditions, opening new avenues for various applications, including sensing, random number generation, and machine learning. Polaritons, hybrid light-matter particles resulting from the strong coupling of photons with excitons in microcavities, present a particularly promising candidate for all-optical systems. Their unique properties allow them to collect and amplify light in a manner that inherently supports a normal noise distribution, which is essential for diffusion models.
Members of HeIsingberg Pathfinder consortium from the University of Cambridge have proposed an innovative all-optical implementation of diffusion models using non-Hermitian optical systems, specifically polariton dyads, as reported in Physical Review Letters. In a polariton dyad, two coupled condensates form with asymmetric populations. When many such dyads are arranged in a lattice, the integrated light intensity from each dyad follows a normal distribution, which is ideal for generating the Gaussian noise required in diffusion models. Inherent biases from system imperfections can be counteracted by manipulating pumping strengths, ensuring a more uniform statistical distribution. These asymmetric dyads, with their macroscopic, unequal occupations and phase differences, can efficiently generate the Gaussian noise necessary for diffusion models in machine learning. Such noise can be generated and transmitted all-optically, reducing the need for digital conversions and enhancing overall efficiency, therefore significantly improving processing speed and energy consumption while opening new frontiers for applying machine learning in various domains.
The future of machine learning diffusion models lies in harnessing the unique properties of optical systems, paving the way for advancements previously unimaginable in the digital domain. The use of polariton dyads in all-optical systems marks a significant step towards more efficient and faster diffusion models, potentially transforming the landscape of artificial intelligence.
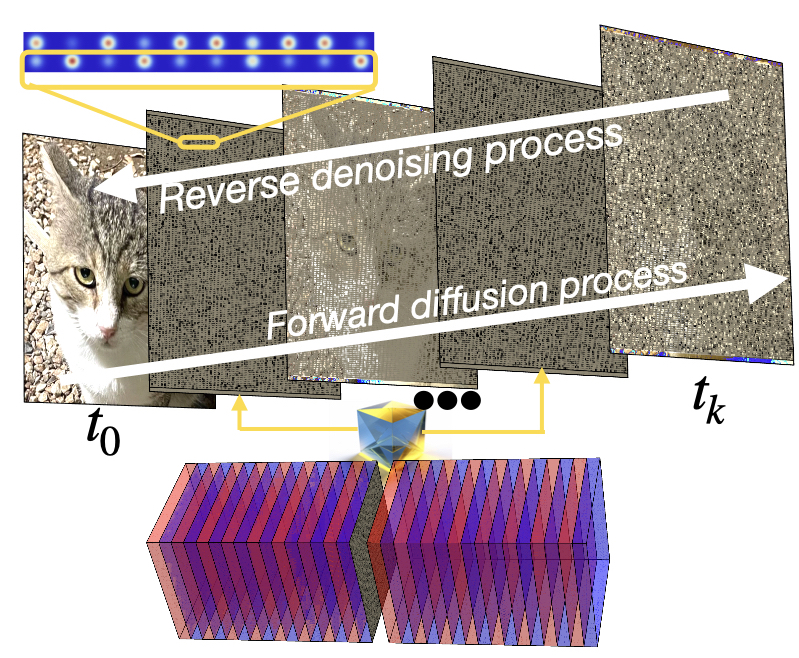
Schematics of the usage of the polariton dyad lattice as optical analog hardware in the generative diffusion process. The lattice of polariton dyads is realised in a microcavity. The emitted light is all-optically guided and superimposed with the pixels of the image at the previous step during the forward diffusion process to transform data into noise gradually. This corresponds to sampling from a normal distribution, while the image gives the mean at the previous step. The reverse process learns to transform the noise back into data.